Ben Kennedy
16 September 2024
Generative Artificial Intelligence (AI) entered the social consciousness in 2022. The release of OpenAI’s GPT-3 and ChatGPT brought Generative AI into the global spotlight, leading to speculation about its impact on knowledge workers, including lawyers. Discussions about its impact on our lives and work are only intensifying.1 The technology’s ability to evaluate text and create new content may have the potential to unlock value for businesses and customers. It is increasingly being integrated into both consumer products, such as phones and social media, and professional tools, including eDiscovery software.2
To gain insight into how our local legal market is evaluating this emerging technology, I recently met with nearly a dozen Australian law firms to hear about their experiences and observations. However, many firms remain hesitant to explore how Generative AI can be practically applied in litigation. There is no consensus on the technology’s immediate value in eDiscovery and a limited understanding of its use's associated (real) procedural risks.
At Adio (a litigation support company), we have been evaluating Generative AI for an increasing list of specific tasks. We’ve achieved several notable outcomes on litigation projects for clients. Typically, we have enriched data to make it more usable, reduced timeframes for specific review tasks, and reduced the hours spent on document management tasks. However, there were also several scenarios where traditional approaches were proven to be more effective than attempting to augment them with Generative AI.
This article explores the practical benefits and challenges of using Generative AI in litigation, particularly in eDiscovery, and examines the procedural risks practitioners must navigate.
Background
The term Generative AI emerged with the release of GPTs (Generative Pre-trained Transformers) built on advanced machine learning techniques involving deep neural networks (large clusters of computers designed to perform many sophisticated and complex computations). Often described as 'next-word predictors’, GPTs generate text through layers of analysis and predict strings of 'tokens' (words or word fragments) likely to follow in a sequence. These models, known as Large Language Models (LLMs), are trained on billions of tokens using various techniques, including learning from human feedback.
There is no database the models query for information. The models have no concept of what is factually true. These models statistically predict the next word in a sequence, producing content that is often coherent and contextually relevant.3
There has been much discussion on the impact of the technology on office work. There are specific examples, admittedly usually promoted by LLM providers, demonstrating where the technology reduced laborious and boring code upgrade tasks from fifty days to a few hours.4 Some studies suggest that up to 50% of tasks in 19% of jobs may be enhanced or replaced by Generative AI, with lawyers and legal secretaries being specifically mentioned as roles that will be impacted.5 AI assistance could be of particular value in litigation where there are court-imposed deadlines, voluminous documents to read and analyse, and limited resources.
When initially considering if this technology is suitable for use in eDiscovery, there is some fundamental ground to cover about how Generative AI works, the security of the service providers, and whether client data will be kept confidential. Jon Tredennick and William Webber’s book Generative AI for Smart People addresses these fundamental questions and provides a helpful overview of the technology for lawyers.6
Australian & New Zealand Court Acceptance
Some high-profile cases in the U.S. have seen judges challenge the unverified use of Generative AI in legal documents.7 Perhaps in response, Australian and New Zealand legal institutions have begun issuing guidance on the appropriate use of Generative AI:
- The NSW Bar Association provided guidance on the considerations for barristers before they use publicly available Generative AI.8
- Victorian courts have issued guidance on the responsible use of AI in litigation and note that “where appropriate, the use of AI should be disclosed to other parties and the court… [and the use of] Generative AI does not relieve the responsible legal practitioner of the need to exercise judgment and professional skill in reviewing the final product to be provided to the Court”.9
- Queensland Courts have provided guidelines for the responsible use of Generative AI for non-lawyers, and while noting “[the technology] may be able to help you prepare some basic legal documents”, the guidance outlines the limitations of Generative AI “Chatbots” and that they should not be relied on as your main source of legal information.10
- New Zealand Courts have provided guidance to staff, lawyers and participants, reinforcing their duties and obligations to the court if the technology is used. The guidance also makes clear that the use of Generative AI does not necessarily need to be disclosed unless asked by the courts.11
- The New Zealand Law Society provides some guidance on Generative AI and suggests that it may be useful in eDiscovery, analysing contracts, legal research, and predicting case outcomes.12 However, the practice note provides that the key to managing the potential risks is understanding how Generative AI works and its limitations, being clear on the use and your obligations, and ensuring there are clear processes for managing confidentiality and privacy, among other things.
These guidelines indicate that while the use of Generative AI is permitted, it must be used cautiously, with particular attention to accuracy, completeness, potential AI 'hallucinations' (false outputs), and security. Rather than issue a directive not to use the technology, the institutions provide clear signposts for responsible and accountable use and acknowledge the potential benefits for both lawyers and clients. The signposts also point towards the risks that should be considered and balanced with the technology's anticipated value.
Navigating the Growing Landscape of AI Models
There has been a rapid evolution of LLMs since 2022. Each new release seems to have an improved capability, usually measured by comparing each model’s performance in multiple choice and written exams in various fields, from medicine to law.13
There are several commercially available “frontier models” from Anthropic, OpenAI, Google, X, and others. These models are expanding beyond the capabilities of other models in specific areas i.e. new frontiers. Open-source models are becoming as capable as their commercial counterparts.14 There is also a trend for releasing smaller and highly capable versions of larger models that run at a fraction of the cost.15 This rapid release of new and improved Generative AI models presents a challenge in selecting the model best suited for specific tasks.
For several reasons, aligning a LLM’s capabilities with a task is fundamental. There is little benefit to be derived from using an LLM to analyse financial documents and review mathematical calculations. LLMs are built to predict the next word, not to identify accounting anomalies or to perform math. The models are often very capable of assessing text for topics and themes of interest.
However, at the time of finishing this article, Open AI released a preview of their reasoning model, o1. The model can effectively undertake a “chain of reasoning” to think through the answers to a problem. The model has math capabilities and overcomes some known limitations of LLMs. OpenAI provides “The models spend more time thinking through problems before they respond, much like a person would. Through training, they learn to refine their thinking process, try different strategies, and recognize their mistakes”.16 If model capabilities continue to evolve, the tasks a model can undertake will increase.
Beyond a LLM’s inherent capabilities, there are several design, implementation and usage decisions that impact their efficacy, including but not limited to:
- Model Parameters can influence how a model responds. For example, model "temperature" adjustments can modulate the model's creativity, making the model's responses more suited to particular tasks. Another important parameter is how large documents are chunked and fed to the model to accommodate the model’s text limits and improve comprehension.
- Prompt engineering is crucial to maximising an LLM's effectiveness. By carefully crafting prompts— such as dictating output formats, stepping through the elements the model should consider in the answer, asking the model to adopt a specific persona, or providing examples—users can significantly improve the model's comprehension of the question and the relevance of its responses.17 Simplicity in prompts seems key; straightforward, focused questions yield better results than complex or multifaceted ones. The words we use matter.18
- Retrieval Augmented Generation (RAG) enables a model to reference specific documents or text outside of their training data. This provides an ability to analyse and refer to documents from a database. However, because RAG relies on secondary indexing, not all content may be referenced, which can obscure nuance.
- Fine-tuning and transfer learning techniques allow additional data and/or layers to be included in the LLM training.19 This process adjusts the model's bias towards the newly introduced content. These techniques require time and effort to build, although they are far less resource-intensive than building a foundational model from scratch.
Despite these techniques to optimise LLM performance, real-world applications still face significant hurdles, as highlighted by a recent Harvard study on using Generative AI in the legal domain. The study found that the inherent difficulty with legal research, the model’s lack of understanding of precedent and case authority, and its tendency to agree with the user (an issue identified as AI Sycophancy), all contributed to hallucinations and incomplete answers in 16% or more scenarios, even with techniques, like RAG.20
Debates over which model and implementation perform best are inevitable. Metrics derived from a model's ability to pass structured exams do not necessarily reflect each model's effectiveness at a task. As such, it is prudent to consider how we measure the technology's performance to demonstrate its appropriate and defensible use for a given task in eDiscovery.
Measuring the performance of Generative AI
Data scientists and institutions are actively reviewing how to measure LLM capabilities and performance.21 Several statistical approaches assess the relevance and diversity of LLM responses, but these metrics alone are insufficient to gauge overall model effectiveness.22 Even the creators of ChatGPT, OpenAI, face challenges in establishing standardised benchmarks. For example, in the release notes of a recent GPT-4o update, OpenAI acknowledged that “Although we’d like to tell you exactly how the model responses are different, figuring out how to granularly benchmark and communicate model behavior improvements are an ongoing area of research in itself.”23 This challenge is relevant in eDiscovery, where accurately assessing a model’s performance is core to defensively using generative AI in tasks like document review.
There are two key metrics that measure search efficacy, ‘Precision’ and ‘Recall’. Precision measures the proportion of retrieved documents that are relevant, while Recall measures the proportion of all relevant documents that are retrieved. These metrics have been foundational for evaluating Technology Assisted Review (TAR) workflows in eDiscovery.
In TAR workflows, machine learning models are trained on samples of documents within a database.24 The model ranks each document based on the characteristics they share with known relevant and irrelevant ones. Documents that share many characteristics with relevant documents receive a high score (e.g., close to 100) while sharing characteristics of irrelevant documents produces a low score (e.g., close to 0).
For example, when evaluating documents with a score above 95, the model might return nine relevant documents out of fifteen and include one irrelevant document, giving a Precision of 90% and Recall of 60%. If we instead evaluate documents scoring above 75, the model might return twelve relevant and six irrelevant documents, reducing Precision to 66% but increasing Recall to 80%. This highlights the inherent trade-off between Precision (accuracy) and Recall (completeness), where higher Recall often means lower Precision, and vice versa.
TAR workflows are generally implemented in two main ways: TAR 1.0 and TAR 2.0. In TAR 1.0, the system is trained upfront using sample documents, and Precision and Recall are assessed at intervals until a desired performance level is achieved. Once reached, no further training occurs. TAR 2.0, by contrast, is a dynamic approach where the system continuously learns from documents as they are reviewed, adjusting rankings, Precision, and Recall as more relevant documents are identified. After either approach, a validation exercise is often conducted to confirm the effectiveness of the process.25
On the other hand, LLM-driven workflows are approached differently. Instead of analysing document characteristics across a dataset, LLMs begin with prompts (crafted questions about a specific topic) designed to guide the model in assessing the relevance of individual documents. These prompts do not evolve dynamically. The prompts remain static unless actively adjusted. As such, the LLM performs a document-by-document assessment based on these prompts, not by identifying relevant and irrelevant characteristics across the entire dataset, as TAR does.
Given this, there is merit in combining LLM and TAR-like technologies. TAR’s strength lies in its ability to identify relevant document characteristics, while LLMs offer flexibility with intuitive, tailored prompts to assess the wording within individual documents independent of other characteristics. In practice, the LLM can assess a document that exhibits relevant characteristics but is irrelevant according to the specific prompts.
For example, if contracts within a particular date range are of interest, TAR algorithms may flag all contracts as relevant. The LLM can then further refine this, identifying which contracts fall within the specified dates of interest.
Finally, while Precision and Recall remain important, they may not be the only means of assessing the performance of TAR and LLM workflows. A recent study suggests that Recall is not always the best measure of TAR performance, arguing that a technology-assisted review can be considered effective if it is on par with human reviewers as the benchmark.26 This benchmark could serve as a useful guide when integrating LLM-driven review processes.
Calculating the value of Generative AI
Once the LLM’s accuracy is quantified (i.e., how often the model is incorrect in its classification), we can compare its use against the manual equivalent. Typical factors to consider include the time to complete tasks, cost, error rate, the effort to address the errors, the risk associated with errors, etc.
To illustrate how AI-augmented workflows can impact the time and cost of a review effort, consider the following basic example.
Example Invoice Review
The task is to identify the invoice in the list, search for it in the database, and note the reference number on the list.
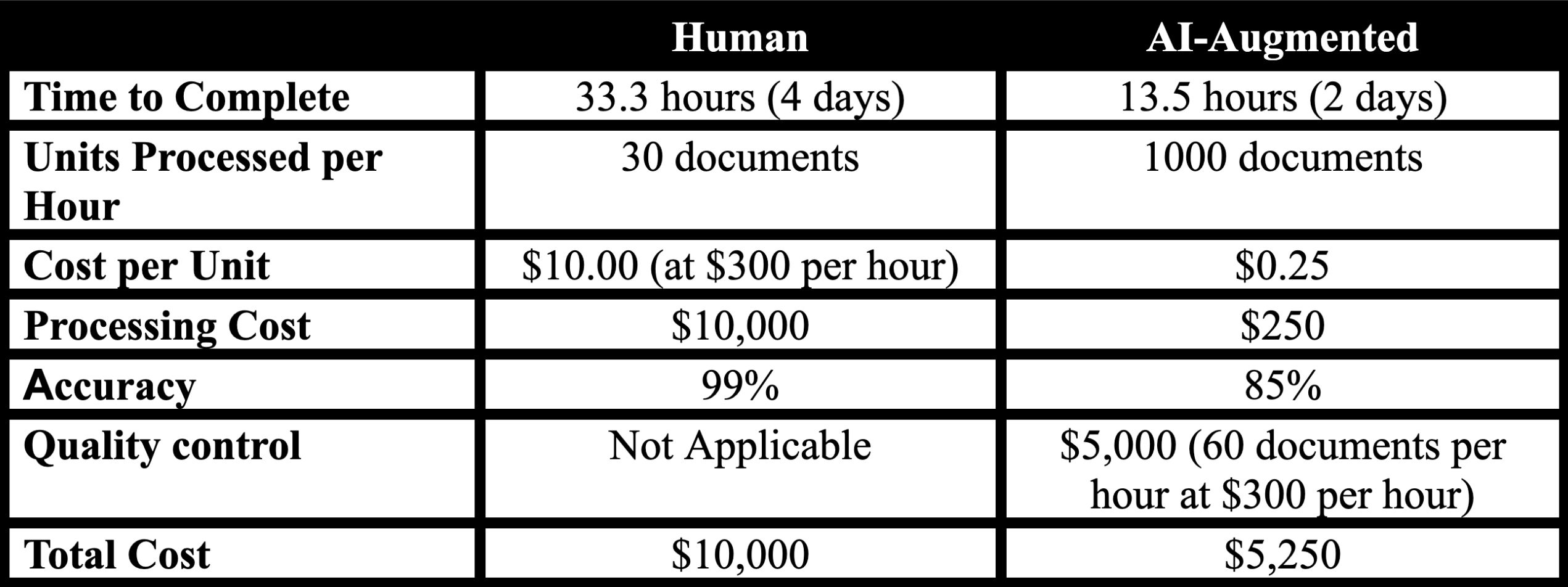
Beyond the immediate cost and time savings shown in this example, implementing AI-augmented workflows can have long-term implications for reducing headcount, improving employee satisfaction, and enabling previously cost-prohibitive tasks, etc. An example task in eDiscovery that is likely to be increasingly performed by AI is the extraction of a date from the face of a document, rather than relying on malleable computer metadata to describe the date of the document.
For businesses and teams, direct experience with AI technology helps identify technical challenges, refine workflows, and quantify potential benefits, laying the foundation for broader adoption—even if model capabilities remain as they are today (which is unlikely).27
The Need for Critical Thinking and Sound Judgement
Generative AI’s reasoning and fluency capabilities have great potential for analysing and synthesising evidence. The technology can answer our questions about the data, summarise documents, build timelines, streamline memo drafting, and more. While these capabilities can enhance productivity and consistency, the uncertainty about whether Generative AI captures all relevant information (completeness) and produces accurate outputs (truthfulness) can still lead to hesitation and inefficiency.
One study of professional consultants showed a 43% improvement in average worker performance and a 16% boost in skilled worker productivity when tasks aligned with the AI's capabilities.28 However, the study also found that when AI was used for tasks beyond its scope, inefficiencies increased by 14-24%, resulting in lower-quality work.29
For legal teams, a misalignment between AI capabilities and tasks can lead to wasted time and resources, particularly when attempting to 'force-fit' the model to complex legal analysis or nuanced interpretation of case law. Lawyers must be prepared to act quickly when the technology doesn’t provide value and is being used for tasks beyond its capabilities. Ultimately, the key is knowing when AI enhances efficiency and when human expertise is irreplaceable.
Adopting Generative AI: A Balanced Approach
Microsoft CTO Kevin Scott suggests that AI is on an exponential improvement curve, with each iteration realising new possibilities every few years.30 However, even with current capabilities, Generative AI can provide value to legal teams.
Structured data extraction and summarisation are well-suited to LLMs. We believe the use of LLMs for these tasks in eDiscovery will be a standard within twelve months, like how we make images text searchable today. Even if not perfect, the output is superior to relying on file system metadata.
With the appropriate level of commitment, Generative AI can be augmented with tasks where humans undertake the same task in parallel, such as document review and classification. Notwithstanding, traditional metrics of Precision and Recall are still relevant in gauging the overall efficacy of the search. Additional approaches to measuring AI performance against a human control group may be an additional means of proving the defensible use of the technology.
Generative AI has the potential to accelerate tasks like evidence analysis and narrative creation. As models evolve in reasoning capabilities, the tools are expected to bring more value to analytical tasks. The AI's accuracy, completeness, and truthfulness in these tasks should be verified and checked. Open-minded but critical-thinking lawyers can use this technology to draft and ideate well-researched and considered advice.
According to McKinsey, Generative AI could unlock up to $4.4 trillion of economic value globally.31 Businesses are beginning to move from testing the technology to scaling it with a view to improving customer experiences and their bottom line.32 There is immense opportunity for legal practitioners to align with their clients’ journeys by integrating AI technology into their practice, so long as the guideposts are observed and the risks managed.
Adio
www.adio.au
1 Gen AI’s next inflection point: From employee experimentation to organizational transformation, 7 August 2024, https://www.mckinsey.com/capabilities/people-and-organizational-performance/our-insights/gen-ais-next-inflection-point-from-employee-experimentation-to-organizational-transformation.
2 Everlaw https://www.everlaw.com/blog/ai-and-advanced-analytics/introducing-everlaw-ai-assistant/, Disco https://csdisco.com/pressrelease/disco-launches-cecilia-auto-review, Relativity https://www.aap.com.au/aapreleases/cision20240625ae46137/, Reveal https://aimresearch.co/generative-ai/reveal-launches-ask-a-game-changing-generative-ai-tool-for-the-legal-sector.
3 For an interactive overview of how transformers work, see Transformer Explainer, https://poloclub.github.io/transformer-explainer/, sourced 2 September 2024.
4 Andy Jassy LinkedIn post on the time savings derived from using their own LLM Amazon Q https://www.linkedin.com/posts/andy-jassy-8b1615_one-of-the-most-tedious-but-critical-tasks-activity-7232374162185461760-AdSz/?utm_source=share&utm_medium=member_ios, 28 August 2024
5 GPTs are GPTs: An Early Look at the Labor Market Impact Potential of Large Language Models, 17 March 2023, https://arxiv.org/abs/2303.10130.
6 Generative AI for Smart People, Tredennick and Dr. Webber, June 2024, https://www.merlin.tech/genai-book-download/.
7 USA, v. Michael Cohen, SDNY, 20 March 2024 https://casetext.com/case/united-states-v-cohen-213 , Mata v Avianca, SDNY, 22 June 2023, https://www.courthousenews.com/wp-content/uploads/2023/06/chatGPT-sanctions-ruling.pdf.
8 Issues Arising from the Use of AI Language Models (including ChatGPT) in Legal Practice, NSW Bar Association, July 2023, https://inbrief.nswbar.asn.au/posts/9e292ee2fc90581f795ff1df0105692d/attachment/NSW%20Bar%20Association%20GPT%20AI%20Language%20Models%20Guidelines.pdf.
9 Guidelines for litigants: responsible use of artificial intelligence in litigation, Supreme Court of Vic., May 2024, https://www.supremecourt.vic.gov.au/forms-fees-and-services/forms-templates-and-guidelines/guideline-responsible-use-of-ai-in-litigation.
10 The Use of Generative Artificial Intelligence (AI) Guidelines for Responsible Use by Non-Lawyers, Queensland Courts, 13 May 2024, https://www.courts.qld.gov.au/__data/assets/pdf_file/0012/798375/artificial-intelligence-guidelines-for-non-lawyers.pdf.
11 Guidelines for use of generative artificial intelligence in Courts and Tribunals, Courts of New Zealand, 7 December 2024, https://www.courtsofnz.govt.nz/going-to-court/practice-directions/practice-guidelines/all-benches/guidelines-for-use-of-generative-artificial-intelligence-in-courts-and-tribunals/.
12 Lawyers and Generative AI, New Zealand Law Society, March 2024, https://www.lawsociety.org.nz/assets/Professional-practice-docs/Rules-and-Guidelines/Lawyers-and-AI-Guidance-Mar-2024.pdf.
13 Figure 1 highlights the performance across 26 examples of GPT 3.5, GPT 4 and GPT 4 (no vision). GPTs are GPTs: An Early Look at the Labor Market Impact Potential of Large Language Models, 22 August 2023, https://arxiv.org/abs/2303.10130.
14 Thanks in large part to companies like Meta taking a free and open-source approach to building models. Although industry benchmarks are not defined, there are some capability comparisons available https://www.edopedia.com/blog/llama-3-1-vs-gpt-4-benchmarks/, sourced 3 September 2024.
15 See OpenAI GPT4o mini https://openai.com/index/gpt-4o-mini-advancing-cost-efficient-intelligence/ and Anthropic Claude 3 Sonnet https://www.anthropic.com/news/claude-3-5-sonnet, sourced 3 September 2024.
16 Introducing OpenAI o1-preview, https://openai.com/index/introducing-openai-o1-preview/, sourced 16 September 2024
17 Prompt Engineering Full Guide, https://daveai.substack.com/p/prompt-engineering-full-guide, sourced 2 September 2024.
18 Words Matter Tips on effective Prompts to Improve Your Generative Output, https://edrm.net/2024/02/words-matter-tips-on-effective-prompts-to-improve-your-generative-ai-output/, sourced 15 August 2023.
19 What is fine-tuning, IBM, 15 March 2024, https://www.ibm.com/topics/fine-tuning.
20 AI on Trial: Legal Models Hallucinate in 1 out of 6 (or More) Benchmarking Queries, 23 May 2024, https://hai.stanford.edu/news/ai-trial-legal-models-hallucinate-1-out-6-or-more-benchmarking-queries.
21 The Electronic Discovery Reference Model, a global advisory on electronic discovery and information governance, has Generative AI working group. Seen on https://edrm.net/edrm-projects/generative-ai/ . The National Institute of Standards and Technology is looking to evaluate model performance as well as detection on where Generative AI has been used to create content. https://ai-challenges.nist.gov/genai sourced 21 August 2024.
22 Perplexity measures how well a language model predicts a sequence of words, BLEU (Bilingual Evaluation Understudy) evaluates how closely the output resembles the Ground Truth and ROUGE (Recall-Oriented Understudy for Gisting Evaluation) metrics are used for evaluating summarisation and translation scores are https://medium.com/data-science-in-your-pocket/llm-evaluation-metrics-explained-af14f26536d2 and https://medium.com/data-science-at-microsoft/evaluating-llm-systems-metrics-challenges-and-best-practices-664ac25be7e5, sourced 21 August 2024.
23 Model Release Notes, OpenAI ChatGPT, 12 August 2024, https://help.openai.com/en/articles/9624314-model-release-notes.
24 Common algorithms used in TAR include Support Vector Machine and Logistic Regression algorithms, and more advanced approaches adopt Bidirectional Encoder Representations from Transformers trained on a specific document corpus.
25 Electronic Discovery Reference Model, Technology Assisted Review Guidelines, January 2019.
26 Unbiased Validation of Technology-Assisted Review for eDiscovery, July 2024, https://dl.acm.org/doi/10.1145/3626772.3657903.
27 Confronting impossible futures, 22 July 2024, https://www.oneusefulthing.org/p/confronting-impossible-futures.
28 Navigating the Jagged Technological Frontier: Field Experimental Evidence of the Effects of AI on Knowledge Worker Productivity and Quality, 22 September 2024, https://mitsloan.mit.edu/sites/default/files/2023-10/SSRN-id4573321.pdf.
29 Ibid.
30 Highlights from an interview with Kevin Scott https://x.com/BasedBeffJezos/status/1818776446199910557, sourced 1 August 2024.
31 The economic potential of generative AI: The next productivity frontier, 14 June 2023, https://www.mckinsey.com/capabilities/mckinsey-digital/our-insights/the-economic-potential-of-generative-ai-the-next-productivity-frontier#industry-impacts.
32 Moving from potential to performance, Deloitte, August 2024, https://www2.deloitte.com/us/en/pages/consulting/articles/state-of-generative-ai-in-enterprise.html.
Interview multiple candidates
Lorem ipsum dolor sit amet, consectetur adipiscing elit proin mi pellentesque lorem turpis feugiat non sed sed sed aliquam lectus sodales gravida turpis maassa odio faucibus accumsan turpis nulla tellus purus ut cursus lorem in pellentesque risus turpis eget quam eu nunc sed diam.
Search for the right experience
Lorem ipsum dolor sit amet, consectetur adipiscing elit proin mi pellentesque lorem turpis feugiat non sed sed sed aliquam lectus sodales gravida turpis maassa odio.
- Lorem ipsum dolor sit amet, consectetur adipiscing elit.
- Porttitor nibh est vulputate vitae sem vitae.
- Netus vestibulum dignissim scelerisque vitae.
- Amet tellus nisl risus lorem vulputate velit eget.
Ask for past work examples & results
Lorem ipsum dolor sit amet, consectetur adipiscing elit consectetur in proin mattis enim posuere maecenas non magna mauris, feugiat montes, porttitor eget nulla id id.
- Lorem ipsum dolor sit amet, consectetur adipiscing elit.
- Netus vestibulum dignissim scelerisque vitae.
- Porttitor nibh est vulputate vitae sem vitae.
- Amet tellus nisl risus lorem vulputate velit eget.
Vet candidates & ask for past references before hiring
Lorem ipsum dolor sit amet, consectetur adipiscing elit ut suspendisse convallis enim tincidunt nunc condimentum facilisi accumsan tempor donec dolor malesuada vestibulum in sed sed morbi accumsan tristique turpis vivamus non velit euismod.
“Lorem ipsum dolor sit amet, consectetur adipiscing elit nunc gravida purus urna, ipsum eu morbi in enim”
Once you hire them, give them access for all tools & resources for success
Lorem ipsum dolor sit amet, consectetur adipiscing elit ut suspendisse convallis enim tincidunt nunc condimentum facilisi accumsan tempor donec dolor malesuada vestibulum in sed sed morbi accumsan tristique turpis vivamus non velit euismod.